How Data Processing is Influential for ML and AI Algorithms?
Data processing tools are becoming increasingly more influential for companies working in Machine learning (ML) and Artificial Intelligence (AI). This is because of its vital effectiveness. Due to the steady expansion of data quantity, dealing with it becomes a daunting task. This is why data wrangling services have become crucial for many companies that deal with non-standard or non-structured data. Artificial intelligence and Machine Learning have been trending for a long time now. The application of these algorithms in all kinds of software is beneficial for almost any business. Especially in the field of sales, the implementation of these methods ensures a solid increase in productivity, sales, and profit. When you think that AI and ML are such young fields of computer science, it will be interesting to know how far they have come.
As we all know, data is the new oil. And as you’ve probably heard it a million times, companies that can utilize data are far more likely to become successful than those who cannot. Data processing is one of the most important components in machine learning. Data preparation and data streaming are equally important to the success of all machine learning projects, with the right data preparation, you can build an efficient, scalable, and accurate model.
How data processing is important for machine learning (ML) and artificial intelligence (AI) algorithms?
Data processing teaches machine learning and artificial intelligence algorithms how to work properly. After extracting structured, semi-structured, and unstructured data, it must be transformed into a form that ML systems can interpret. But relevancy is more important here. You can't expect your ML algorithms to learn what makes them smart and valuable to your business if the data is irrelevant.
Stages of Data Processing
Data Collection
This process entails gathering data from reputable sources and then picking the highest-quality elements of the collection. It's important to remember that quality over quantity is important here. The other aspect to examine is the task's objective.
Data Pre-Processing
Preprocessing implies converting data into an algorithm-friendly format.
- Formatting - Data can be obtained in a variety of formats, including proprietary and Parquet file formats. Data formatting enables learning models to work efficiently with data.
- Cleansing - During this step, you will delete any unnecessary data and will also resolve any instances of missing data.
- Sampling - This stage is critical for maximizing efficiency and memory capacity. Instead of using the entire dataset, you can utilize a smaller sample to explore and prototype solutions faster.
Data Transformation
The algorithm used and the solution sought influence the preprocessed data transformation process. After you've uploaded the dataset to the library, the transformation process begins. Below are just a few of the numerous options.
Levelling - Scaling is the adjustment of numeric variables' values to fit a certain scale like 0 - 1 or 0 - 100. As a result of this technique, the data we acquire are similar and have no odds.
Breakdown - This process converts a heterogeneous model into a triple data model. The transformation rules in this section will classify the data set as structured, semi-structured, or unstructured. Following that, we can select the category that best fits our model's machine learning method.
Aggregation - The raw dataset is aggregated to locate, extract, transfer, and normalize it. This method may be repeated to produce aggregated data that can be kept or used for various purposes. This step has a direct effect on the software system's quality.
Data Interpretation
There are many ways to get meaningful data and it can come in many different forms. An example of this could be a graph, video, report, image, or audio file, because of its compatibility. In the past, the data was encoded for the ML algorithm, but now it is in a form that can be read. Being stored in multiple locations, the data can be accessed by anyone at anytime.
Data Storage
The final stage of the entire process is the step in which data or metadata is stored for future reference.
Data processing is the process of collecting and organizing data before it can be analyzed. Machine learning and artificial intelligence systems can use processed data to anticipate or recommend. Data processing and management are fundamentally important to the functioning of many areas of modern technology, including machine learning and artificial intelligence. Data scientists and engineers must not only design effective algorithms but also ensure that they can be applied effectively in the real world.
Being one of the best data processing companies, BPO Data Entry Help offers the affordable BPO services. With our contemporary strategies, we offer the finest business solutions. To know more, contact us at [email protected]
Recent Post
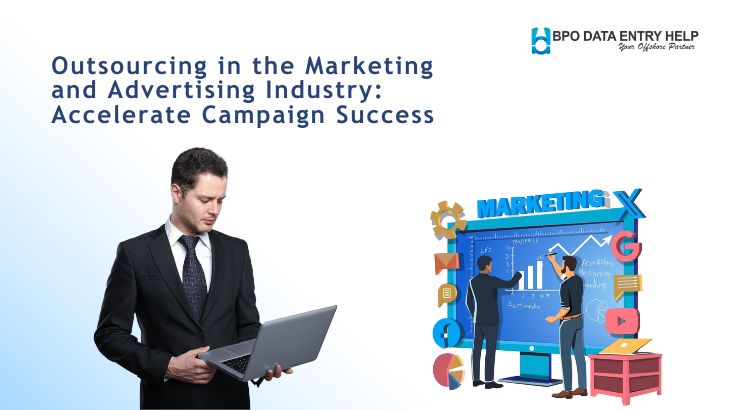
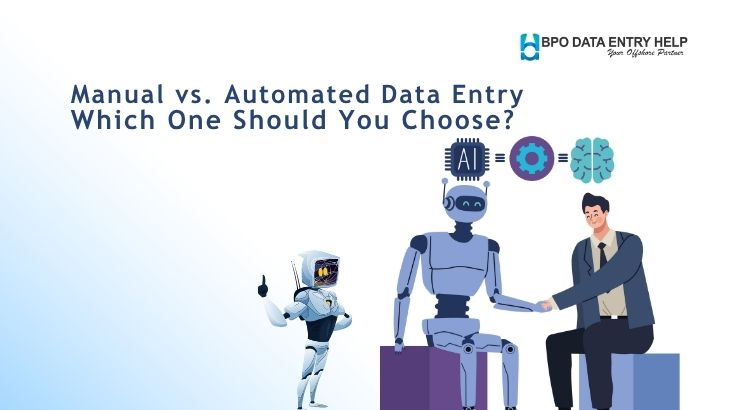
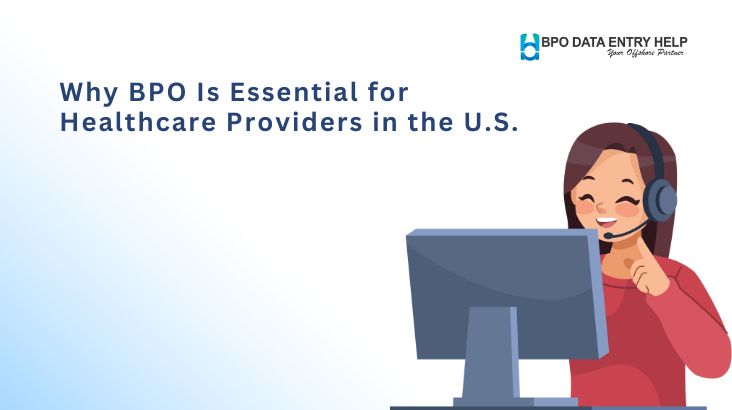
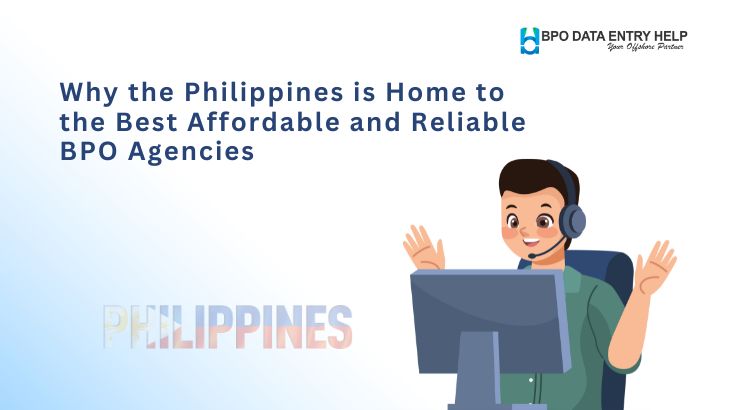
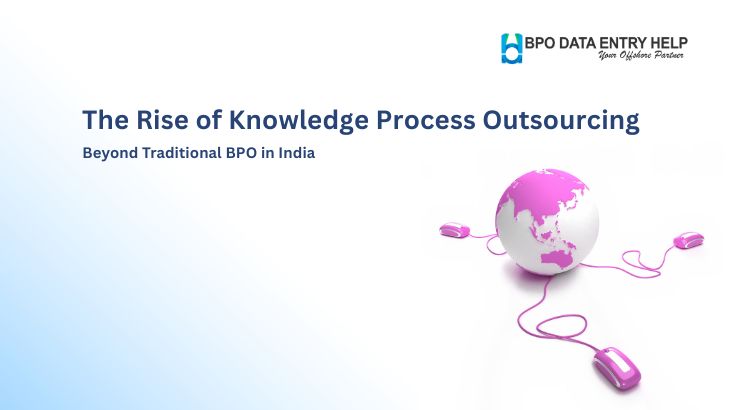